Sponsoring Organizations
|
2017 ACC Plenary and
Semi-Plenary Lectures
The conference technical, plenary and semi-plenary, and
special sessions will reflect the diversity of theory and applications
of control that is one of the hallmarks of an ACC.
Our slate of plenary and semi-plenary speakers consists of well-known
researchers and leaders from academia.
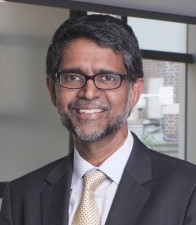 |
Plenary Lecture
Flying Robots: Towards Smaller, Safer, Smarter and Faster UAVs
Professor Vijay Kumar
University of Pennsylvania, USA
ABSTRACT The number of unmanned aerial vehicles or
drones has grown exponentially over the last three decades. Yet we
are only now seeing autonomous flying robots that can operate in
three-dimensional indoor environments and in outdoor environments
without GPS. I will discuss the need for smaller, safer, smarter, and
faster flying robots and the challenges in control, planning, and
coordinating swarms of robots with applications to search and rescue,
first response and precision farming. Publications and videos are
available at
kumarrobotics.org.
BIOGRAPHY
Vijay Kumar is the Nemirovsky Family Dean of Penn
Engineering with appointments in the Departments of
Mechanical Engineering and Applied Mechanics, Computer
and Information Science, and Electrical and Systems
Engineering at the University of Pennsylvania. Dr. Kumar received his
Bachelor of Technology degree from the Indian
Institute of Technology, Kanpur and his Ph.D. from The Ohio State
University in 1987. He has been on the Faculty in the Department of
Mechanical Engineering and Applied Mechanics with a secondary
appointment in the Department of Computer and Information Science at the
University of Pennsylvania since 1987.
He served as the Deputy Dean for Research in the School of
Engineering and Applied Science, and directed the GRASP
Laboratory, a multidisciplinary robotics and perception laboratory from 2000-2004.
He was the Chairman of the Department of Mechanical
Engineering and Applied Mechanics from 2005-2008. He served as the
Deputy Dean for Education in the School of Engineering and Applied
Science from 2008-2012. He then served as the assistant director of
robotics and cyber physical systems at the White House Office of Science
and Technology Policy (2012 – 2013). Dr. Kumar is a Fellow of the American Society of Mechanical Engineers
(2003), a Fellow of the Institute of Electrical and Electronic Engineers
(2005) and a member of the National Academy of Engineering (2013).
Dr. Kumar’s research interests are in robotics, specifically multi-robot
systems, and micro aerial vehicles. He has served on the editorial
boards of the IEEE Transactions on Robotics and Automation, IEEE
Transactions on Automation Science and Engineering, ASME Journal of
Mechanical Design, the ASME Journal of Mechanisms and Robotics and the
Springer Tract in Advanced Robotics (STAR).
He is the recipient of the 1991 National Science Foundation Presidential
Young Investigator award, the 1996 Lindback Award for Distinguished
Teaching (University of Pennsylvania), the 1997 Freudenstein Award for
significant accomplishments in mechanisms and robotics, the 2012 ASME
Mechanisms and Robotics Award, the 2012 IEEE Robotics and Automation
Society Distinguished Service Award , a 2012 World Technology Network
Award, and a 2014 Engelberger Robotics Award. He has won best paper
awards at DARS 2002, ICRA 2004, ICRA 2011, RSS 2011, and RSS 2013, and
has advised doctoral students who have won Best Student Paper Awards at
ICRA 2008, RSS 2009, and DARS 2010.
|
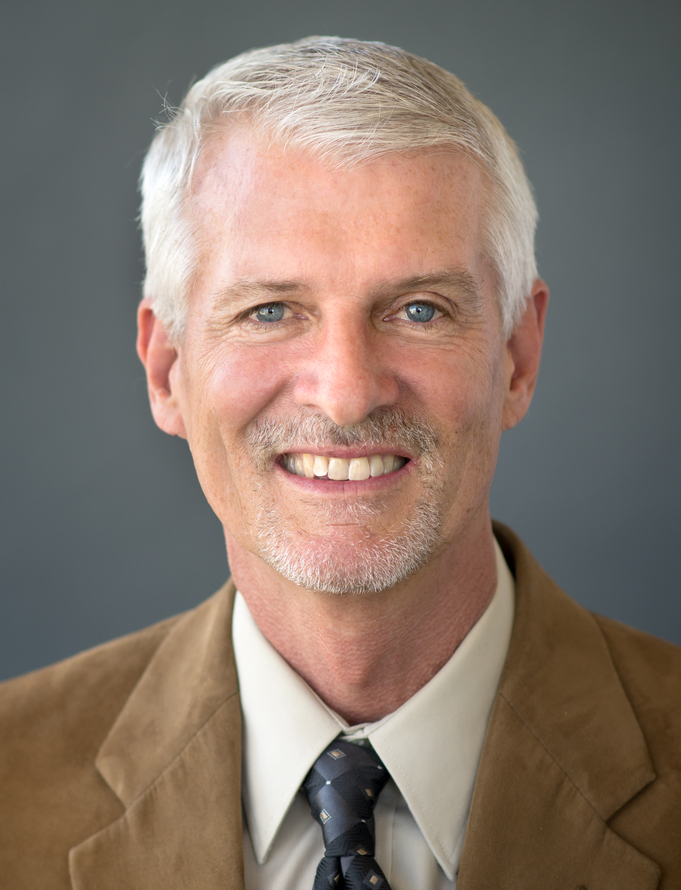 |
Semi-Plenary Lecture
Achieving High Level Control Goals with Model Predictive Control
Professor James Rawlings
University of Wisconsin, USA
ABSTRACT Model predictive control has become a pervasive advanced
control technology in which optimal control of a multivariable system
with input and state constraints is combined with a moving horizon to
produce a feedback controller. In applications, model
predictive control is often used to solve constrained tracking
problems. The tracking problem arises in some settings as the basic
goal of the control system, and the constraint handling capabilities
of MPC are what make it attractive. In other applications, however,
there may be a higher-level goal, such as economic optimization of a
process, and this goal is first translated into a steady-state
tracking problem. Since MPC enables the designer to choose the
objective function that is optimized online, it offers the potential
to treat the higher-level control goal directly within the MPC
controller bypassing this translation into a steady-state setpoint and
tracking problem.
In this talk we explore the possibilities enabled by MPC to address
these types of high-level goals. We also outline some of the open
research challenges presented by this approach; these include
modeling, optimization, and controller design challenges. The talk
concludes with a brief presentation of a recently deployed economic
optimization technology developed by Johnson Controls to control the
campus energy system at Stanford University.
BIOGRAPHY
James B. Rawlings received the B.S. from the University of Texas
and the Ph.D. from the University of Wisconsin, both in Chemical
Engineering. He spent one year at the University of Stuttgart as a
NATO postdoctoral fellow and then joined the faculty at the University
of Texas. He moved to the University of Wisconsin in 1995 and is
currently the Steenbock Professor of Engineering and W. Harmon Ray Professor of
Chemical and Biological Engineering, and the co-director of the
Texas-Wisconsin-California Control Consortium (TWCCC).
Professor Rawlings's research interests are in the areas of chemical
process modeling, monitoring and control, nonlinear model predictive
control, moving horizon state estimation, and molecular-scale chemical
reaction engineering. He has written numerous research articles and
coauthored three textbooks: "Modeling and Analysis Principles for
Chemical and Biological Engineers" (2013) with Mike Graham, "Model
Predictive Control: Theory and Design" (2009), with David Mayne, and
"Chemical Reactor Analysis and Design Fundamentals," 2nd ed. (2012),
with John Ekerdt.
In recognition of his research and teaching, Professor Rawlings has
received several awards including:
National Academy of Engineering;
"Doctor technices honoris causa" from the Danish Technical
University;
The inaugural High Impact Paper Award from the International
Federation of Automatic Control;
The Ragazzini Education Award from the American Automatic Control
Council;
The Computing in Chemical Engineering Award and Excellence in Process
Development Award from the AICHE;
The Chancellor's Distinguished Teaching Award, a WARF Named
Professorship, and the Byron Bird Award for Excellence in a Research
Publication, from the University of Wisconsin;
He is a fellow of IFAC, IEEE, and AIChE.
|
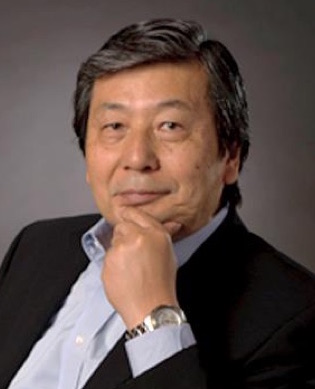 |
Semi-Plenary Lecture
A Data-driven Approach to Nonlinear Systems Control, Robotics,
and Life Sciences in the Era of Big Data
Professor Harry Asada
Massachusetts Institute of Technology (MIT), USA
ABSTRACT
Data-driven techniques are increasingly important as a
vast amount of data is becoming available at low cost.
Yet, effective methodologies are still lacking for
extracting critical information needed for control
design from large data sets. This talk will address how
we can exploit Big Data, both measured and simulated,
for complex system modeling and control. First, a new
data-driven approach to building an effective state
equation will be developed by using Latent Variable
methods combined with physical modeling theory, the Bond
Graph. Two major features and challenges will be
addressed. One is to find a complete set of variables
that can sufficiently inform the system’s nonlinear
dynamics. Independent state variables are augmented by
adding auxiliary variables that are needed for
describing constitutive laws of individual components,
which may be nonlinear. The other is to show that a
class of nonlinear dynamical systems behaves linearly
when it is recast in a high-dimensional space derived
from the augmented state space that is sufficiently
informative. While the resultant latent state equation
is linear, complex nonlinearities are embedded in the
compact model, leading to precise and global
linearization of nonlinear dynamics. The new methodology
will be applied to: a) Model Predictive Control of
nonlinear systems, b) global stable control of
underwater robots subject to nonlinear hydrodynamics,
including ground effect and nonlinear drag, and c)
intracellular and intercellular biochemical dynamics of
interacting cells. These examples demonstrate that
linearity of the augmented state equations allows for a)
eliminating complex nonlinear stochastic prediction, b)
supernumerary state feedback for assuring global
stability, and c) superposition of multiple solutions to
predict emergent behaviors of interacting nonlinear
agents, which would otherwise be prohibitively complex
to compute.
BIOGRAPHY
H. Harry Asada is Ford Professor of Engineering and
Director of the Brit and Alex d’Arbeloff Laboratory for
Information Systems and Technology in the Department of
Mechanical Engineering, Massachusetts Institute of
Technology, Cambridge, MA. He received his B.S., M.S.,
and Ph.D. degrees in precision engineering in 1973,
1975, and 1979, respectively, all from Kyoto University,
Japan. He specializes in robotics, biological
engineering, and system dynamics and control. His
current research includes precise, global linearization
of nonlinear systems, wearable extra legs, arms, and
fingers, underwater robots, bio-artificial muscles,
optogenetic control, computational modeling of cell
migration and emergent behaviors. He received the Rufus
Oldenburger Medal from ASME in 2011. He won the Best
Paper Awards at the IEEE International Conference on
Robotics and Automation in 1993, 1997, 1999, and 2010,
the O. Hugo Schuck Best Paper Award from the American
Control Council in 1985, Best Journal Paper Awards from
the Society of Instrument and Control Engineers in 1979,
1984, and 1990, and the Henry Paynter Outstanding
Researcher Award from ASME Dynamic Systems and Control
in 1998. He also received the Spira Award for
Distinguished Teaching from the School of Engineering,
MIT. Dr. Asada is a Fellow of ASME.
|
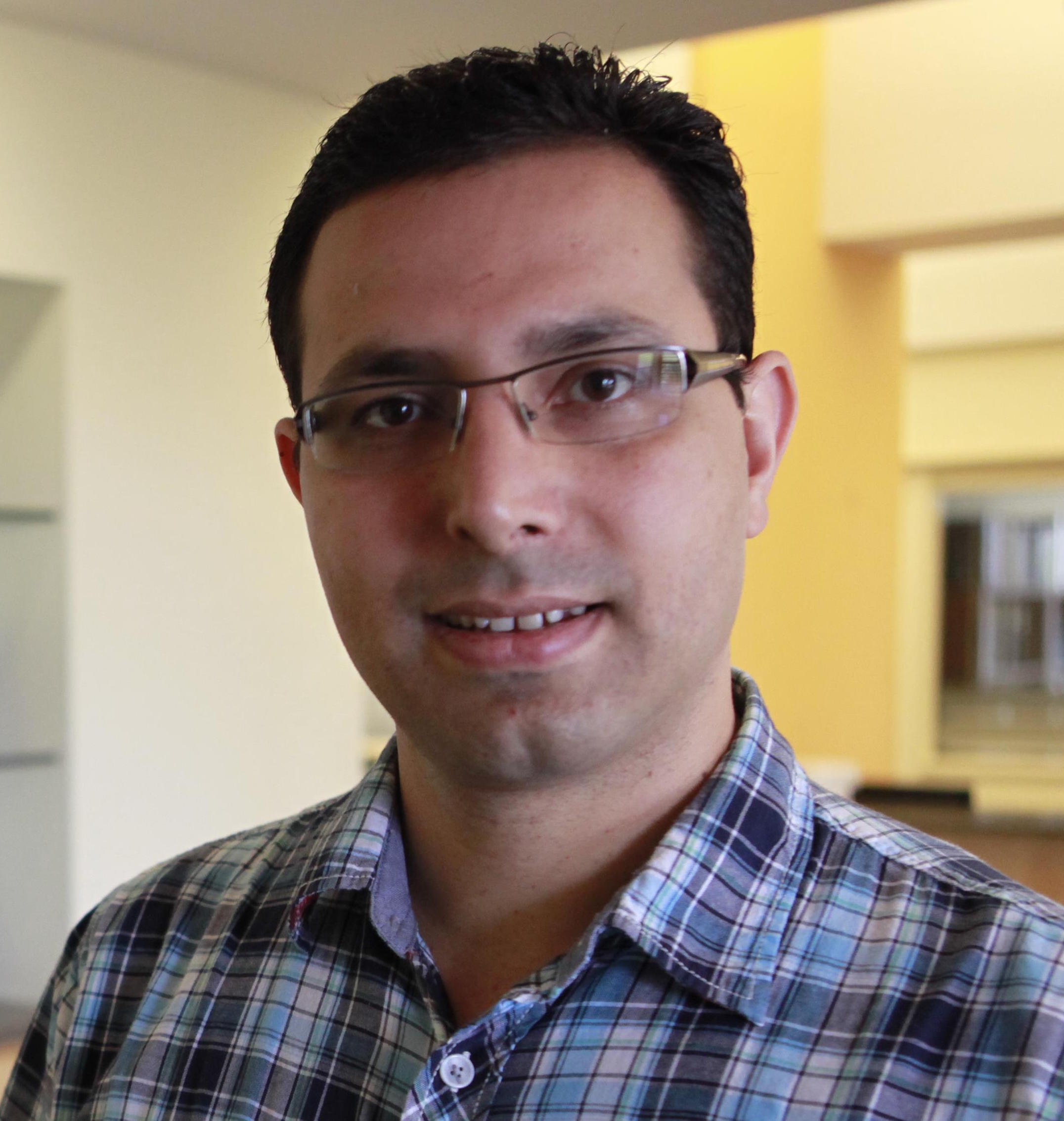 |
Semi-Plenary
Lecture
Graph-Theoretic Convexification of Polynomial
Optimization Problems with Applications to Power Systems
and Distributed Control
Professor Javad Lavaei (2016 Eckman Award Winner)
University of California, Berkeley, USA
ABSTRACT
The area of polynomial optimization has been
actively studied in computer science, operations
research, applied mathematics and engineering, where the
goal is to find a high-quality solution using an
efficient computational method. This area has attracted
much attention in the control community since several
long-standing control problems could be converted to
polynomial optimization problems. The current researches
on this area have been mostly focused on various
important questions: i) how does the underlying
structure of an optimization problem affect its
complexity? Ii) how does sparsity help? iii) how to find
a near globally optimal solution whenever it is hard to
find a global minimum? iv) how to design an efficient
numerical algorithm for large-scale non-convex
optimization problems? v) how to deal with problems with
a mix of continuous and discrete variables? In this
talk, we will develop a unified mathematical framework
to study the above problems. Our framework rests on
recent advances in graph theory and optimization,
including the notions of OS-vertex sequence and
treewidth, matrix completion, semidefinite programming,
and low-rank optimization. We will also apply our
results to two areas of power systems and distributed
control. In particular, we will discuss how our results
could be used to address several hard problems for power
systems such as optimal power flow (OPF),
security-constrained OPF, state estimation, and unit
commitment.
BIOGRAPHY
Javad Lavaei is an Assistant Professor in the
Department of Industrial Engineering and Operations
Research at University of California, Berkeley. He was
an Assistant Professor in Electrical Engineering at
Columbia University from 2012 to 2015. He received the
Ph.D. degree in Control & Dynamical Systems from the
California Institute of Technology in 2011, and was a
postdoctoral scholar in Electrical Engineering and
Precourt Institute for Energy at Stanford University for
one year. He is the recipient of the Milton and Francis
Clauser Doctoral Prize for the best university-wide
Ph.D. thesis, entitled "Large-Scale Complex Systems:
From Antenna Circuits to Power Grids". He researches on
optimization theory, control theory and power systems.
He has won several awards, including DARPA Young Faculty
Award, Office of Naval Research Young Investigator
Award, National Science Foundation CAREER Award,
Resonate Award, Google Faculty Research Award, Governor
General of Canada Academic Gold Medal, Northeastern
Association of Graduate Schools Master's Thesis Award,
and Silver Medal in the 1999 International Mathematical
Olympiad. Javad Lavaei is an associate editor of IEEE
Transactions on Smart Grid and serves on the conference
editorial board of IEEE Control Systems Society and
European Control Association. He was a finalist (as an
advisor) for the Best Student Paper Award at the 53rd
IEEE Conference on Decision and Control 2014. His
journal paper entitled "Zero Duality Gap in Optimal
Power Flow Problem" has received a prize paper award
given by the IEEE PES Power System Analysis Computing
and Economics Committee in 2015. He is a co-recipient of
the 2015 INFORMS Optimization Society Prize for Young
Researchers, and the recipient of the 2016 Donald P.
Eckman Award given by the American Automatic Control
Council |
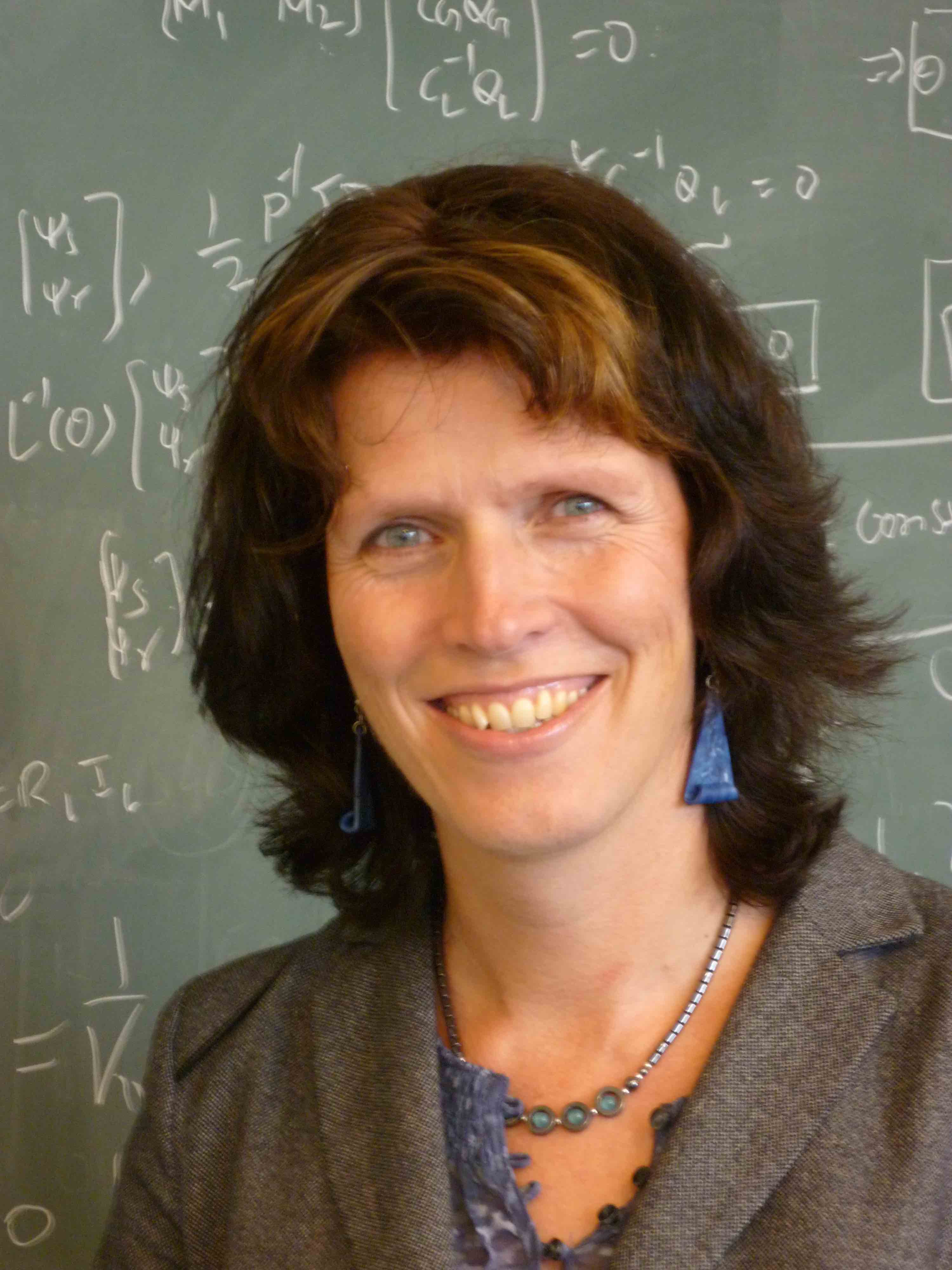 |
Semi-Plenary Lecture
Model Reduction of Networks Preserving the Network First and Second Order Structure
Professor Jacquelien Scherpen
University of Groningen, The Netherlands
ABSTRACT
Network systems have received a lot of attention in
the past decade. They are used to analyze and design
communication network, smart grid technology, social
media, social dynamics, formation and consensus
problems, etc. Several analysis and control methods have
been developed for network systems. However, often,
their large scale nature makes it difficult to analyze
and to design a controller. We develop methods to reduce
the order of the network while preserving the network
structure, as well as some structure of the (linear)
node dynamics. In particular, second order network
dynamics structure is preserved. We use node clustering
methods, as well as a state space singular value
decomposition based method. For the first we provide
error bounds. We illustrate the results with help of
some relevant high order examples.
BIOGRAPHY
Jacquelien M. A. Scherpen received the M.Sc. and
Ph.D. degrees in applied mathematics from the University
of Twente, Enschede, The Netherlands, in 1990 and 1994,
respectively. She was with Delft University of
Technology, The Netherlands, from 1994 to 2006. Since
September 2006, she is a professor at the University of
Groningen, at the Engineering and Technology institute
Groningen (ENTEG) of the Faculty of Mathematics and
Natural Sciences, The Netherlands. Since 2013 she is the
scientific director of ENTEG. She is a member of the
Jan C. Willems Center for Systems and Control of the
University of Groningen, and board member of the Dutch
Institute of Systems and Control. She has held visiting
research positions at the University of Tokyo, and
Kyoto University, Japan, Université de Compiegne, and
SUPELEC, Gif-sur-Yvette, France, and the Old Dominion
University, Norfolk, VA, USA. She has been an Associate
Editor of the IEEE Transactions on Automatic Control,
the International Journal of Robust and Nonlinear
Control (IJRNC) and the IMA Journal of Mathematical
Control and Information. She is on the editorial board
of the IJRNC. Her current research interests include
(linear and nonlinear) model reduction methods,
nonlinear control methods, modeling and control of
physical systems using the concepts of passivity and
dissipativity, and distributed optimal control
applications for smart energy system.
|
|
|
|
Key Dates
Draft Manuscripts:
Monday September 19, 2016
Best Student Paper Nominations:
Friday, September 30, 2016
Workshop Proposals:
Monday, October 10, 2016
Acceptance/Rejection Notice:
Sunday, January 22, 2017
Final Manuscript Submission:
Tuesday, February 28, 2017
Contacts for Sponsors:
Junmin Wang
wang.1381@osu.edu (Vice Chair for Industry
Activities)
Stefano Di Cairano
dicairano@ieee.org
(Exhibits Chair)
Jing Sun
jingsun@umich.edu
(General Chair)
|